A pre-trained model, whether in the visual or textual domain, refers to a machine learning model that undergoes initial training on a large and diverse dataset before being fine-tuned for specific tasks. In the textual domain, models like OpenAI's GPT (Generative Pre-trained Transformer) are pre-trained on extensive text data from the internet, acquiring a comprehensive understanding of language structure, context, and semantics. Similarly, in the visual domain, models based on architectures like Convolutional Neural Networks (CNNs), such as VGG or ResNet, are pre-trained on vast image datasets to learn general features and representations of visual data, such as edges and textures. This pre-training enables the models to capture broad patterns and knowledge from the data, and they can subsequently be fine-tuned for specific applications, like text generation or image classification, resulting in improved performance on targeted tasks with potentially less task-specific training data.
Why use pre-trained models?Pre-trained models are employed in both the visual and textual domains for their ability to leverage large and diverse datasets during initial training, capturing general patterns and knowledge inherent in the data. In the textual domain, models like GPT learn language nuances, syntactic structures, and contextual relationships, providing a foundation for various natural language processing tasks. In the visual domain, pre-trained models based on CNNs grasp hierarchical features and representations of visual data, facilitating tasks like image classification or object detection. The advantage lies in the transferability of knowledge gained during pre-training to specific tasks, allowing for more effective and efficient learning with potentially reduced amounts of task-specific training data. This approach accelerates model development, enhances performance, and proves particularly valuable when labeled data for a specific task is limited, as the pre-trained models have already acquired a broad understanding of their respective domains.
MAIN WORKEnhancing Plant Disease Diagnosis: Innovative Multimodal Datasets and Pre-trained Models for Improved Accuracy and Efficiency
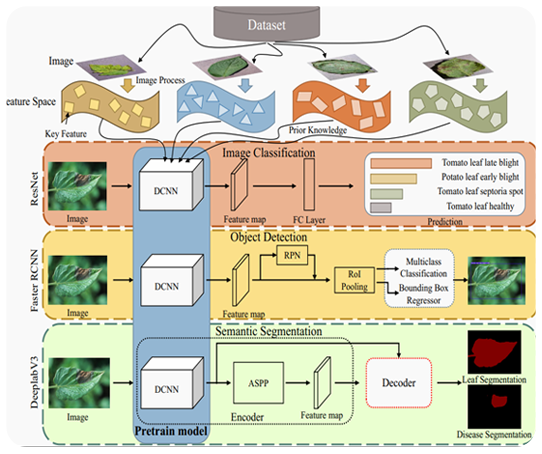
PDDD-PreTrain: A series of commonused pre-trained models support image-based plant disease diagnosis
A large-scale plant disease diagnostic database (PDDD) of more than 400,000 images from 40 plant species and 120 disease categories was collected. Moreover, a series of commonly used visual pre-trained models (PDDD-Pretrain) trained on the PDDD database were designed to improve the performance of plant disease diagnosis. Compared with existing pre-trained models, these plant disease visual pre-trained models can achieve higher accuracy with shorter training time, thus supporting better diagnosis of plant diseases and promoting the intelligent development of plant disease diagnosis. Plant Phenomics, 2023, DOI: 10.34133/plantphenomics.0054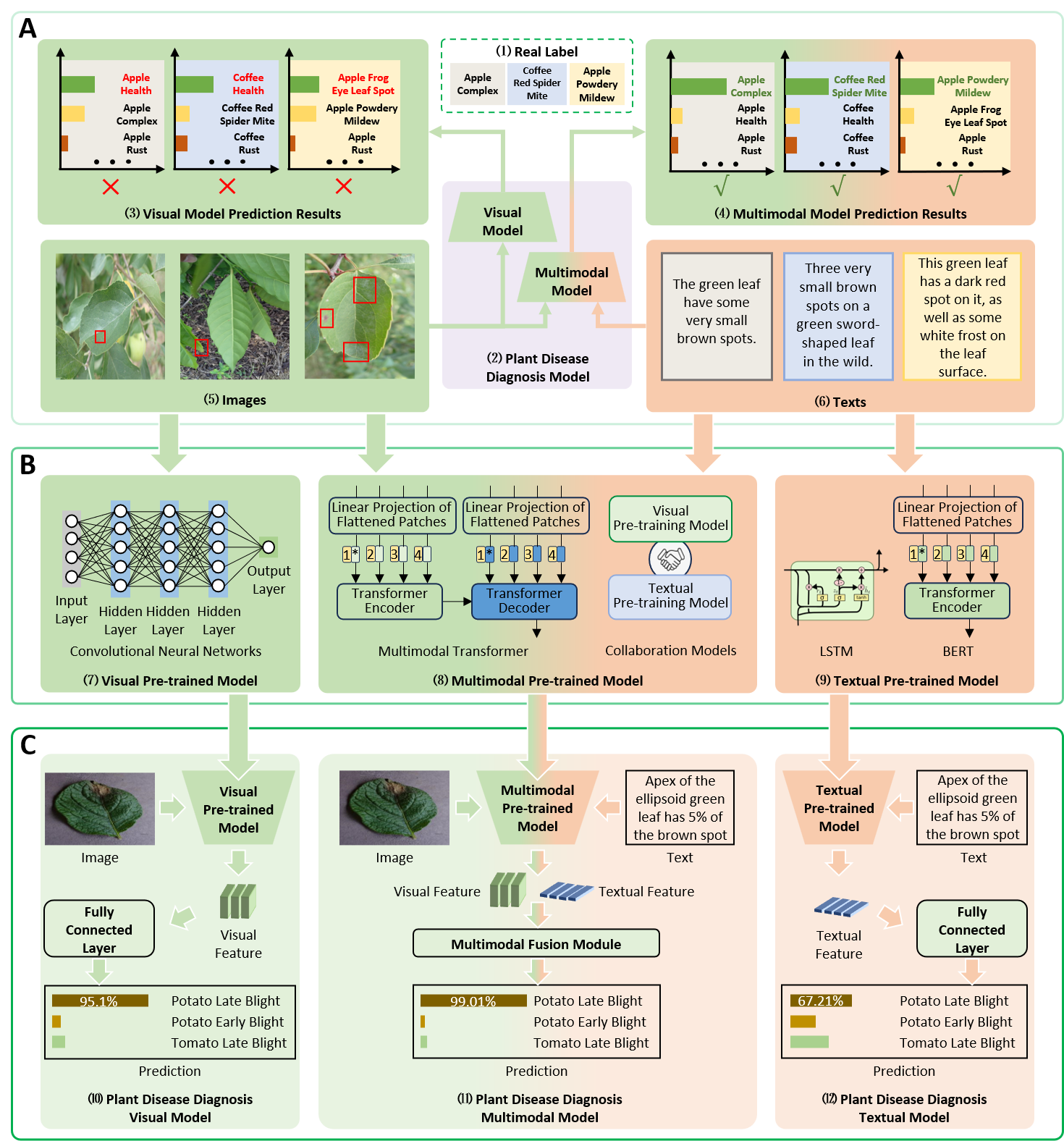
PDDM-Pretrain: Plant Disease Diagnosis Multimodal Pre-trained Model Families
The constructed large-scale plant disease diagnostic multimodal (PDDM) database was used to train a family of multimodal pre-trained models (PDDM-Pretrain) to improve the performance and efficiency of plant disease diagnostic models. The PDDM database has 116 categories of plant diseases, 205,007 images, and 410,014 expert diagnostic texts. PDDM-Pretrain has 22 visual pre-trained models, 4 text pre-trained models and 4 multimodal pre-trained models. These pre-trained models can provide a large amount of a priori knowledge of visual semantics and textual semantics of plant diseases for plant disease diagnosis models.